Auxiliary variables
Auxiliary variables are variables that can help to make estimates on incomplete data, while they are not part of the main analysis (Collins et al., 2001). These variables are related to the probability of missingness in a variable and/or related ot the incomplete variable itself. By including auxiliary variables in a missing data analyses, the reason for missingness in a missing at random situation and extra information about the incomplete values is taken into account.
In regression based imputation methods, as single (stochastic) regression imputation, but also in multiple imputation methods, auxiliary variables can be included in the model for imputation. These extra variables help estimating the imputed values and can increase precision and decrease bias. The figure below presents the role of auxiliary variables in such a model respective to the variables in the analysis.
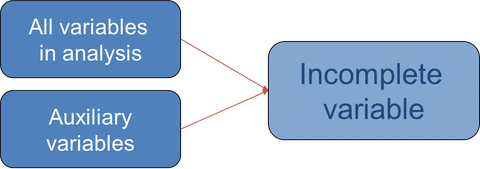
In missing data methods that are likelihood based, as the structural equation models estimated by full information maximum likelihood, the inclusion of auxiliary variables is done through the covariance matrix in the estimation of the models. Shafer (2003) describes several methods to include the auxiliary variables in these kinds of models. The figure below displays the inclusion of two auxiliary variables in a simple structural equation model. The auxiliary variables should be directly correlated to the measured predictor variables, and to the error of the outcome variable. The auxiliary variables should also be correlated with each other.
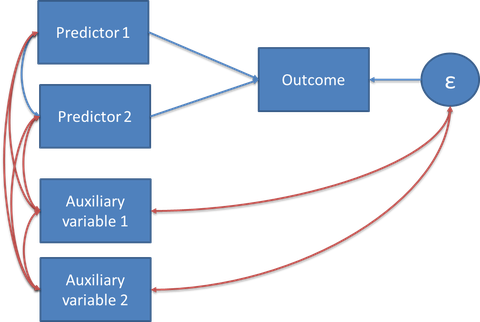